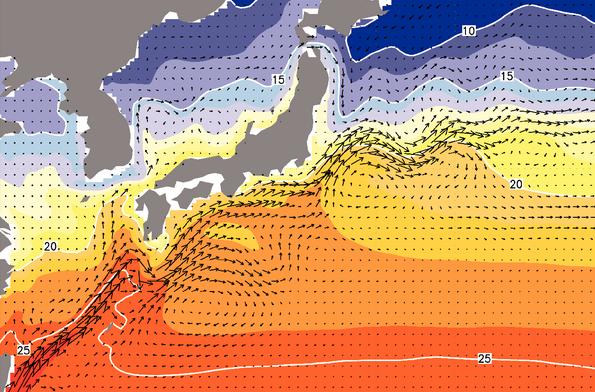
Talk to someone who rejects the conclusions of climate science and you’ll likely hear some variation of the following: “That’s all based on models, and you can make a model say anything you want.” Often, they'll suggest the models don't even have a solid foundation of data to work with—garbage in, garbage out, as the old programming adage goes. But how many of us (anywhere on the opinion spectrum) really know enough about what goes into a climate model to judge what comes out?
Climate models are used to generate projections showing the consequences of various courses of action, so they are relevant to discussions about public policy. Of course, being relevant to public policy also makes a thing vulnerable to the indiscriminate cannons on the foul battlefield of politics.
Skepticism is certainly not an unreasonable response when first exposed to the concept of a climate model. But skepticism means examining the evidence before making up one’s mind. If anyone has scrutinized the workings of climate models, it’s climate scientists—and they are confident that, just as in other fields, their models are useful scientific tools.
It’s a model, just not the fierce kind
Climate models are, at heart, giant bundles of equations—mathematical representations of everything we’ve learned about the climate system. Equations for the physics of absorbing energy from the Sun’s radiation. Equations for atmospheric and oceanic circulation. Equations for chemical cycles. Equations for the growth of vegetation. Some of these equations are simple physical laws, but some are empirical approximations of processes that occur at a scale too small to be simulated directly.
Cloud droplets, for example, might be a couple hundredths of a millimeter in diameter, while the smallest grid cells that are considered in a model may be more like a couple hundred kilometers across. Instead of trying to model individual droplets, scientists instead approximate their bulk behavior within each grid cell. These approximations are called “parameterizations.”
Connect all those equations together and the model operates like a virtual, rudimentary Earth. So long as the models behave realistically, they allow scientists to test hypotheses as well as make predictions testable by new observations.
Some components of the climate system are connected in a fairly direct manner, but some processes are too complicated to think through intuitively, and climate models can help us explore the complexity. So it's possible that shrinking sea ice in the Arctic could increase snowfall over Siberia, pushing the jet stream southward, creating summer high pressures in Europe that allow India’s monsoon rains to linger, and on it goes… It's hard to examine those connections in the real world, but it's much easier to see how things play out in a climate model. Twiddle some knobs, run the model. Twiddle again, see what changes. You get to design your own experiment—a rare luxury in some of the Earth sciences.
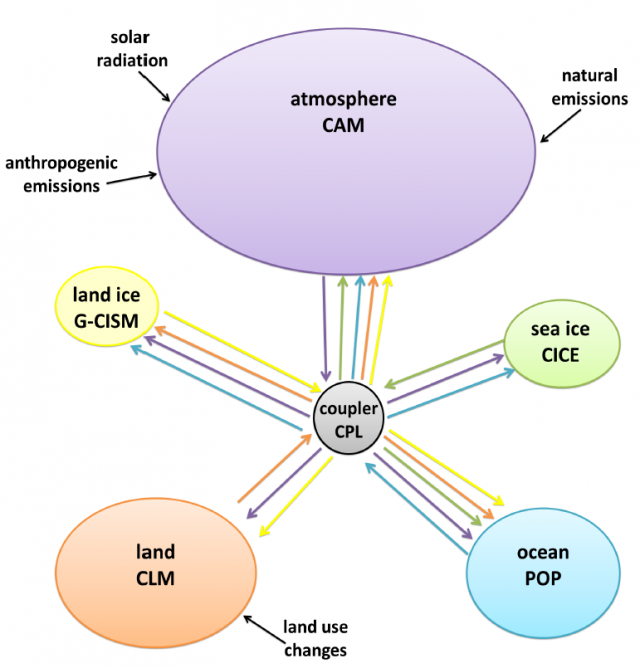
In order to gain useful insights, we need climate models that behave realistically. Climate modelers are always working to develop an ever more faithful representation of the planet’s climate system. At every step along the way, the models are compared to as much real-world data as possible. They’re never perfect, but these comparisons give us a sense for what the model can do well and where it veers off track. That knowledge guides the use of the model, in that it tells us which results are robust and which are too uncertain to be relied upon.
Andrew Weaver, a researcher at the University of Victoria, uses climate models to study many aspects of the climate system and anthropogenic climate change. Weaver described the model evaluation process as including three general phases. First, you see how the model simulates a stable climate with characteristics like the modern day. “You basically take a very long run, a so-called ‘control run,'” Weaver told Ars. “You just do perpetual present-day type conditions. And you look at the statistics of the system and say, 'Does this model give me a good representation of El Niño? Does it give me a good representation of Arctic Oscillation? Do I see seasonal cycles in here? Do trees grow where they should grow? Is the carbon cycle balanced?'”
Next, the model is run in changing conditions, simulating the last couple centuries using our best estimates of the climate “forcings” (or drivers of change) at work over that time period. Those forcings include solar activity, volcanic eruptions, changing greenhouse gas concentrations, and human modifications of the landscape. “What has happened, of course, is that people have cut down trees and created pasture, so you actually have to artificially come in and cut down trees and turn it into pasture, and you have to account for this human effect on the climate system,” Weaver said.
The results are compared to observations of things like changing global temperatures, local temperatures, and precipitation patterns. Did the model capture the big picture? How about the fine details? Which fine details did it simulate poorly—and why might that be?
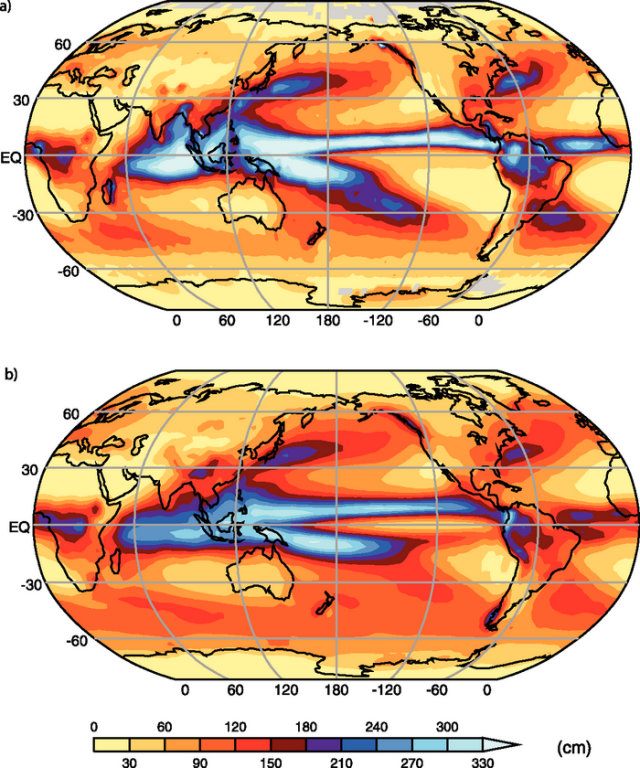
At this point, the model is set loose on interesting climatic periods in the past. Here, the observations are fuzzier. Proxy records of climate, like those derived from ice cores and ocean sediment cores, track the big-picture changes well but can’t provide the same level of local detail we have for the past century. Still, you can see if the model captures the unique characteristics of that period and whatever regional patterns we’ve been able to identify.
This is what models go through before researchers start using them to investigate questions or provide estimates for summary reports like those produced for the Intergovernmental Panel on Climate Change.